Table of Contents
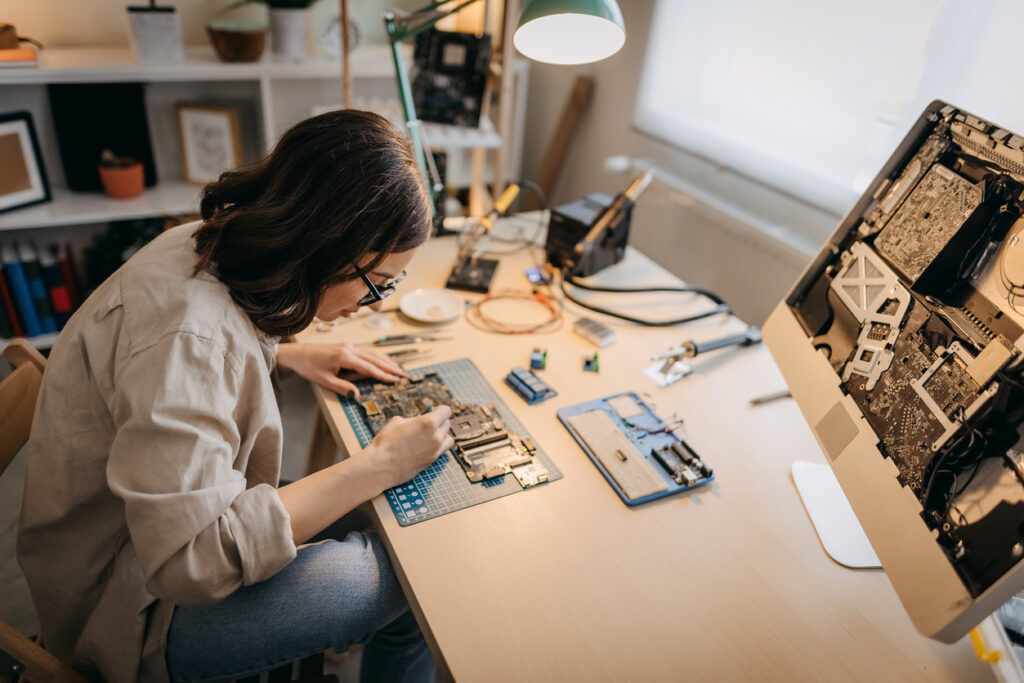
Innovative AI Solutions with AMD GPU Tech
In the rapidly evolving field of artificial intelligence (AI), the role of hardware in driving innovation and efficiency is more crucial than ever. As AI applications become more complex and resource-intensive, the demand for high-performance GPUs has skyrocketed. AMD, a long-standing leader in GPU technology, has made significant strides in this space, particularly with AI-focused solutions that challenge the dominance of industry leader NVIDIA.
This article delves into AMD’s journey in GPU development, highlighting the evolution of its technology and the introduction of innovative architectures designed specifically for AI workloads. From the historic acquisition of ATI Technologies to the launch of cutting-edge accelerators like the MI300X, AMD has consistently pushed the boundaries of what GPUs can achieve in AI. We will explore the key features of AMD’s current and upcoming AI GPU offerings, compare their performance against NVIDIA’s AI GPU counterparts, and discuss the potential benefits of switching to AMD GPUs for AI tasks, particularly in terms of cost-effectiveness, performance, and open-source support.
Uncover the latest trends in AI cloud computing and how to leverage the power of AI.Ebook: Navigating AI Cloud Computing Trends
The Evolution of AMD GPU Technology
Before we delve into AMD’s current offerings, let’s briefly examine the historical perspective on AMD’s journey in GPU development. AMD’s foray into GPU development can be traced back to the early 2000s when they acquired ATI Technologies, a move that significantly bolstered their graphics capabilities. This acquisition provided AMD with a strong foundation in GPU technology and paved the way for future advancements in parallel processing and high-performance computing.
Over the years, AMD has continued to push the boundaries of GPU design, introducing groundbreaking technologies such as HBM (High Bandwidth Memory) and RDNA architecture. These innovations have improved the performance and efficiency of AMD’s GPUs and solidified their reputation as a leading provider of graphics solutions for a wide range of applications, from gaming to data centers. AMD has a long-standing history of innovation in the GPU space, starting with its first release of the ATI Radeon 9700 Pro in 2002. Since then, AMD has consistently pushed the boundaries of GPU technology, introducing several groundbreaking features and performance improvements over the years, making them better candidates for AI tasks.
Key milestones in AMD’s GPU technology evolution include the introduction of unified shader architectures, such as AMD’s Tessellation and Geometry Engines. These advancements significantly enhanced rendering capabilities and paved the way for more realistic and immersive visual experiences.
Another significant development in AMD’s GPU technology timeline is the introduction of High-Bandwidth Memory (HBM) in its graphics cards. HBM revolutionized memory architecture by stacking memory dies vertically, allowing for faster data transmission and reduced power consumption. This innovation improved overall performance and paved the way for more compact and energy-efficient GPU designs.
Overview of Current and Future AMD AI GPUs
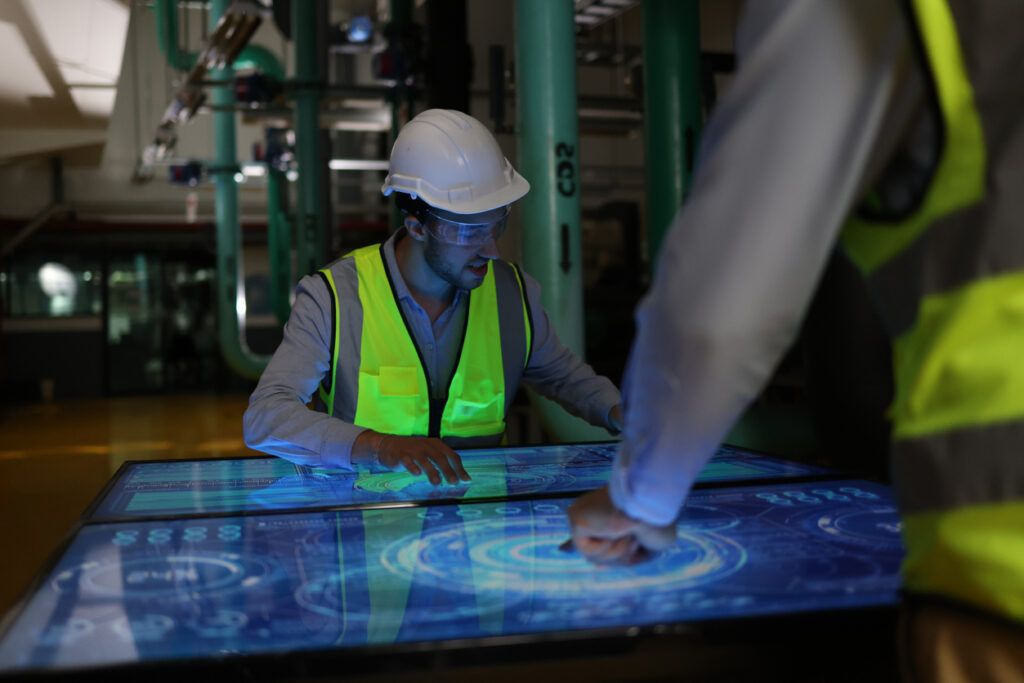
The new AMD GPU lineup offers a diverse range of options tailored to meet various AI workload requirements, catering to different industries and applications. Let’s delve deeper into the innovative offerings so you can choose the best AMD GPU for your needs:
Architecture/Accelerator | Target Market | Key Features | Primary Applications |
AMD CDNA | Data centers, high-performance computing (HPC) |
|
AI workloads in demanding environments, complex matrix calculations |
AMD XDNA | Gaming, content creation |
|
AI-enhanced gaming, real-time rendering, stunning visual fidelity |
AMD Zen | General-purpose computing, AI acceleration |
|
Broad spectrum of AI applications requiring robust computing power |
AMD RDNA | Gaming, content creation |
|
Gaming, graphics-intensive tasks, AI-driven applications |
AMD Instinct MI300X | AI research and development |
|
Accelerating complex AI computations, faster AI insights and breakthroughs |
MI325X Accelerator | AI workloads |
|
Training deep neural networks, running inference tasks, accelerating AI workflows |
AMD CDNA
The AMD CDNA architecture stands out as a powerhouse designed specifically for data center and high-performance computing applications. Boasting cutting-edge features like Matrix Core and Infinity Fabric, CDNA GPUs excel in delivering exceptional compute performance and efficiency. These GPUs are finely tuned to handle complex matrix calculations with remarkable speed and precision, making them a top choice for AI workloads in demanding environments.
AMD XDNA
Targeting the gaming and content creation sectors, the AMD XDNA architecture is engineered to provide high-performance AI capabilities. These GPUs strike a perfect balance between compute and graphics performance, offering a seamless blend that is ideal for powering AI-enhanced gaming experiences and enabling real-time rendering with stunning visual fidelity. The XDNA architecture represents AMD’s commitment to pushing the boundaries of AI technology in consumer-facing industries.
AMD “Zen”
While renowned for its dominance in the CPU market, the AMD Zen architecture also shines in the realm of AI acceleration. Zen-based APUs (Accelerated Processing Units) leverage the combined processing power of CPUs and GPUs to deliver efficient AI performance across a wide spectrum of applications. This fusion of computational prowess and AI capabilities positions Zen-based APUs as versatile solutions for tasks requiring both robust computing power and advanced AI processing.
AMD RDNA
As AMD’s latest graphics architecture tailored for gaming and content creation, the AMD RDNA architecture represents a significant leap forward in visual computing technology. While primarily optimized for gaming and graphics-intensive tasks, RDNA GPUs exhibit remarkable compute performance that enables them to effectively tackle a variety of AI workloads. The versatility of RDNA GPUs makes them a compelling choice for users seeking a well-rounded solution that excels in both gaming and AI-driven applications.
AMD Instinct MI300X Accelerators
In addition to the architectural advancements, AMD’s Instinct MI300X accelerators further enhance AI performance. These accelerators are designed to handle demanding AI workloads with ease and are backed by AMD’s commitment to open-source software.
The AMD Instinct MI300X accelerators are at the forefront of innovation in the AI hardware space. Leveraging cutting-edge technology, these accelerators are optimized to deliver unparalleled performance for a wide range of AI applications. From deep learning to data analytics, the MI300X accelerators excel in accelerating complex computations, enabling faster insights and breakthroughs in AI research and development.
MI325X Accelerator
The AMD Instinct MI325X accelerator is a powerful option tailored specifically for AI workloads. It supports mixed-precision matrix operations, enabling efficient processing of large datasets. With its high-performance compute capabilities, the MI325X accelerator delivers exceptional AI performance.
Equipped with advanced features such as enhanced memory bandwidth and optimized power efficiency, the MI325X accelerator is designed to meet the demanding requirements of modern AI applications. Whether it’s training deep neural networks or running inference tasks, the MI325X accelerator provides the computational power needed to drive AI innovation forward. Researchers, data scientists, and developers can rely on the MI325X accelerator to accelerate their AI workflows and push the boundaries of what is possible in artificial intelligence.
Performance Comparison between AMD and NVIDIA GPUs for AI Tasks
When it comes to choosing the right GPU for AI tasks, the competition between AMD vs. NVIDIA GPUs is steadily increasing. Both companies offer powerful GPUs, but they differ significantly in terms of performance, cost-effectiveness, software support, and ecosystem compatibility. This comparative analysis will delve into these aspects to help you make an informed decision.
Aspect | NVIDIA GPUs | AMD GPUs |
AI Performance | Leader in AI with CUDA and Tensor Cores, excels in deep learning tasks. | Strong in FP32 precision tasks, competitive with ROCm, especially in open-source environments. |
Tensor/Mixed Precision Cores | Tensor Cores significantly accelerate matrix operations, ideal for deep learning. | Lacks equivalent to Tensor Cores, but strong in FP32 precision tasks like image classification. |
Benchmark Results | Dominates benchmarks in AI models like ResNet, BERT, and GPT-3, especially in mixed-precision tasks. | Improving in benchmarks with ROCm, closing the gap in FP32 tasks, outperforming in specific scenarios. |
Real-World Performance | Highly optimized with deep CUDA integration in frameworks like TensorFlow and PyTorch. | Catching up in real-world scenarios but still trails slightly due to a less mature ecosystem. |
Cost-Effectiveness | Generally higher priced, justified by superior performance and efficiency in high-end AI workloads. | More affordable, offering a compelling ROI for cost-sensitive projects and smaller-scale applications. |
ROI | Higher initial investment with quicker ROI due to faster project completions and efficiency. | Lower initial cost with decent performance, offering good ROI for non-critical applications. |
Software & Ecosystem Support | Strong with CUDA/cuDNN, extensive integration with AI frameworks, broad community and resources. | Focus on open-source (ROCm), growing support but still behind NVIDIA in ecosystem maturity. |
1. Performance Comparison for AI Tasks
NVIDIA GPUs:
- Performance in AI Workloads: NVIDIA has been the leader in the AI space, especially with its CUDA architecture and Tensor Cores, which are designed for accelerating deep learning tasks.
- Tensor Cores: These specialized cores significantly speed up matrix operations, which are crucial for AI tasks such as training and inference in deep learning models.
AMD GPUs:
- Performance with ROCm: AMD’s high-end GPUs, like the MI50 and MI100, particularly excel in FP32 precision tasks. The ROCm (Radeon Open Compute) platform has improved AMD’s performance in AI workloads, making them a competitive alternative, especially for those looking for open-source solutions.
- Mixed Precision Performance: While AMD’s GPUs do not yet match NVIDIA’s Tensor Cores in mixed-precision tasks, they have shown strengths in FP32, especially in certain AI applications like image classification, where the MI50 has outperformed NVIDIA’s RTX 2080 Ti in some benchmarks.
2. Benchmark Results and Real-World Performance
NVIDIA:
- Benchmark Dominance: In benchmark tests involving AI models such as ResNet, BERT, and GPT-3, NVIDIA GPUs typically lead, particularly in mixed-precision training. Their ability to handle complex deep learning models quickly is unmatched, particularly in real-world scenarios where time and efficiency are crucial.
- Real-World Performance: The deep integration of CUDA with AI frameworks like TensorFlow and PyTorch enables highly optimized performance, making NVIDIA GPUs the preferred choice for most AI researchers and developers.
AMD:
- Improved Benchmarks with ROCm: AMD has made significant progress with ROCm, which has been shown to close the performance gap in AI benchmarks. Tests have shown that for specific models and use cases, AMD GPUs, such as the MI50, can outperform NVIDIA equivalents in FP32 tasks.
- Real-World Usage: While AMD is catching up in benchmarks, real-world performance can still lag slightly behind NVIDIA due to the maturity and optimization of NVIDIA’s ecosystem. However, for open-source and cost-sensitive applications, AMD offers a strong value proposition.
3. Cost-Effectiveness and Value Proposition
Price Comparison:
- NVIDIA: Generally, NVIDIA GPUs are priced higher than AMD counterparts, reflecting their performance advantages and mature software ecosystem. However, for businesses requiring the best-in-class AI performance, the higher cost is often justified by the faster training times and higher efficiency.
- AMD: AMD GPUs typically offer a lower price point, making them an attractive option for cost-sensitive projects. Their recent advancements in AI performance have made them a viable alternative, particularly for workloads where the highest level of mixed-precision performance is not necessary.
ROI Analysis:
- NVIDIA: For companies focused on large-scale AI projects, the higher initial investment in NVIDIA hardware can lead to a faster return on investment due to quicker project completions and reduced operational costs over time.
- AMD: AMD provides a compelling ROI for smaller projects or those that do not require the absolute highest performance. The lower initial cost, combined with decent AI performance, makes AMD GPUs a cost-effective solution for many organizations.
4. Software and Ecosystem Support
NVIDIA:
- CUDA and cuDNN: NVIDIA’s software stack, including CUDA and cuDNN, is deeply integrated with major AI frameworks like TensorFlow, PyTorch, and Keras. This integration ensures that NVIDIA GPUs are well-optimized for AI tasks out of the box, with extensive support from both the community and the company itself.
- Broad Ecosystem: The dominance of CUDA in the AI field has led to widespread adoption, meaning there is a vast amount of resources, tutorials, and third-party tools available for NVIDIA GPUs.
AMD:
- ROCm and Open-Source Focus: AMD has taken a different approach with ROCm, focusing on open-source software support. While this has made significant strides in recent years, particularly with support for TensorFlow and PyTorch, the ecosystem is not as mature as NVIDIA’s, which can lead to more challenges in optimization and support.
- Compatibility: While AMD’s ROCm is increasingly compatible with popular AI frameworks, the overall ecosystem still trails NVIDIA, particularly in terms of community support and the availability of optimized libraries.
Both AMD and NVIDIA offer compelling options for AI tasks, but the right choice depends on your specific needs:
- Choose NVIDIA if you require top-tier performance, particularly in mixed-precision AI tasks, and need access to a mature, well-supported software ecosystem.
- Choose AMD if you are looking for a cost-effective solution with strong open-source support, especially for workloads that can benefit from FP32 precision.
In essence, NVIDIA remains the gold standard for AI workloads, particularly in high-performance environments, while AMD offers a cost-effective alternative with growing capabilities, especially for users committed to open-source platforms.
Benefits of Switching from for AI
Switching from NVIDIA to AMD GPUs for AI tasks offers several potential benefits, particularly as AMD continues to advance in the AI hardware space. While NVIDIA has long been the leader in AI GPUs, AMD is rapidly closing the gap with competitive offerings that provide distinct advantages.
Key Benefits of Switching to AMD GPUs for AI
1. Cost-Effectiveness
AMD GPUs are typically more cost-effective than NVIDIA’s high-end models. This affordability makes AMD an attractive option for businesses and researchers who require powerful AI hardware but must adhere to budget constraints. By choosing AMD, organizations can scale their cloud AI infrastructure without incurring the high costs often associated with NVIDIA’s offerings. This cost advantage is particularly significant for startups, academic institutions, and companies with limited IT budgets, enabling them to invest in more hardware or allocate resources to other critical areas.
2. Performance Advancements
- Competitive Performance: AMD has been making significant claims about the capabilities of their latest AI accelerators, such as the MI300X. According to AMD, these accelerators can be up to 1.6 times faster than NVIDIA’s top-tier chips, including the NVIDIA H100 SXM. If these performance metrics are validated in real-world AI applications, switching to AMD could offer substantial improvements in processing power, particularly for large-scale AI model training. This enhanced performance positions AMD as a strong competitor in the AI hardware market, capable of delivering the computational horsepower required for advanced AI research and development.
- Support for Larger AI Models: AMD’s upcoming MI300X is designed to handle larger AI models than NVIDIA’s equivalent hardware. This capability is crucial for researchers and data scientists working with complex and resource-intensive AI tasks, such as training deep neural networks on massive datasets. The ability to train larger models more efficiently can lead to faster innovations and breakthroughs in AI, providing AMD users with a competitive edge in cutting-edge research.
3. Open-Source Ecosystem
AMD’s ROCm (Radeon Open Compute) platform underscores the company’s commitment to open-source technologies. ROCm is increasingly compatible with popular AI frameworks like TensorFlow and PyTorch, facilitating easier optimization and integration of AI applications on AMD hardware. For organizations that prioritize open-source development and community-driven innovation, AMD’s ROCm platform offers a flexible and collaborative environment. This open-source focus not only reduces reliance on proprietary software but also fosters a diverse ecosystem where developers can contribute to and benefit from collective advancements.
4. Energy Efficiency
AMD is continuously refining its GPU architectures, with a focus on enhancing energy efficiency. This improvement could translate into lower operational costs, especially in data centers where power consumption is a significant expense. Energy-efficient GPUs are not only cost-effective but also align with sustainability goals, making AMD a strong choice for organizations looking to reduce their environmental footprint while maintaining high-performance AI capabilities.
5. Flexibility in Deployment
AMD GPUs are increasingly being recognized for their flexibility in deployment, particularly in hybrid and multi-cloud environments. As organizations move towards multi-cloud strategies to avoid vendor lock-in and optimize their infrastructure, AMD’s open-source and platform-agnostic approach provides greater flexibility. This makes it easier to integrate AMD GPUs into diverse cloud environments, offering a seamless experience across different cloud providers and on-premises data centers.
6. Support for High-Precision AI Tasks
While NVIDIA excels in mixed-precision tasks with Tensor Cores, AMD has shown strong performance in FP32 precision tasks, which are essential for certain AI workloads that require high accuracy. This capability makes AMD GPUs particularly well-suited for applications like scientific computing, where precision is critical. Organizations focused on these high-precision tasks can benefit from AMD’s ability to deliver consistent and reliable performance in FP32 computations.
While NVIDIA remains a dominant force in the AI GPU market, AMD’s rapidly advancing technology offers a viable and increasingly compelling alternative. The combination of cost-effectiveness, competitive performance, support for larger AI models, commitment to open-source solutions, and potential for improved energy efficiency makes AMD GPUs an attractive choice for a wide range of AI applications. As AMD continues to innovate and challenge NVIDIA’s dominance, they are poised to become a go-to option for AI workloads, especially for organizations looking to balance performance with affordability and flexibility.
Conclusion
As AI continues to transform industries, the need for powerful, efficient, and scalable GPU solutions has never been greater. AMD’s advancements in GPU technology, particularly in AI workloads, present a compelling alternative to NVIDIA’s dominance in the field. With innovations like the MI300X accelerator and a strong commitment to open-source ecosystems, AMD offers both performance and affordability, making it an attractive option for businesses and researchers alike. While NVIDIA remains a benchmark in AI performance, AMD’s rapidly evolving technologies provide a viable and increasingly competitive choice for those looking to harness the power of AI. As the landscape of AI hardware continues to evolve, AMD is poised to become a key player, offering innovative solutions that meet the complex needs of the modern AI-driven world.
Discover how NZO Cloud can help you build an optimized, efficient, and productive cloud environment for your business. Reach out to us today for a free trial.