Table of Contents
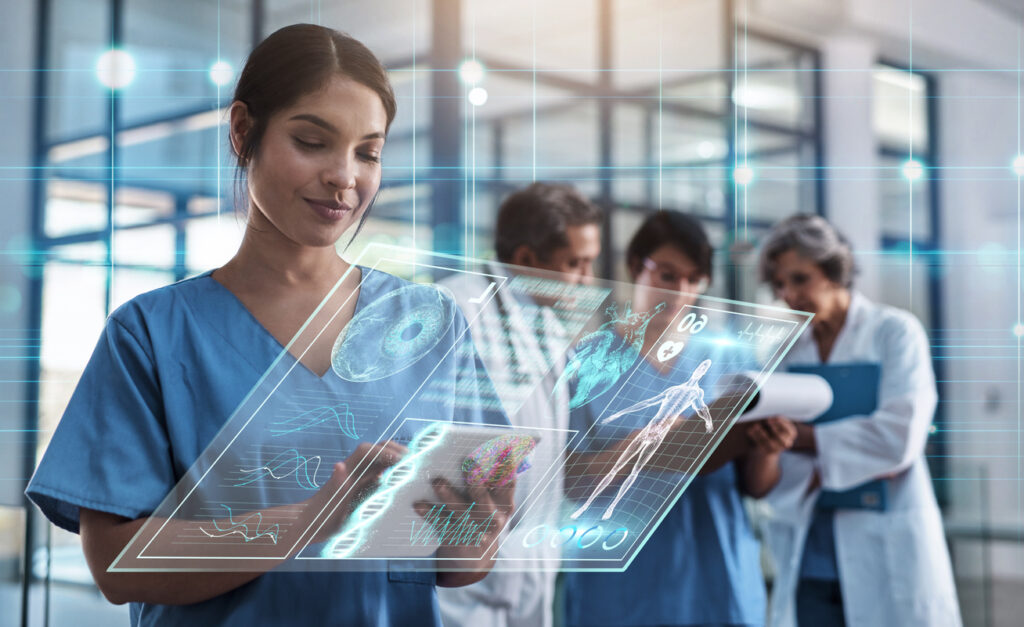
Artificial Intelligence (AI) is reshaping medical imaging, bringing transformative improvements to diagnostics and patient care. Through advanced image analysis, AI systems can quickly identify patterns and abnormalities across a range of medical scans, including X-rays, MRIs, CT scans, and more. By harnessing vast datasets and sophisticated algorithms often on clouds run by government or academic facilities, AI helps radiologists, cardiologists, and other specialists make more accurate, timely diagnoses, often detecting issues that might be missed by relying on the human eye alone.
This AI-driven enhancement in diagnostic accuracy not only accelerates the speed at which conditions are identified but also reduces human error, contributing to early intervention and improved patient outcomes. As a result, AI’s integration into medical imaging is proving to be a crucial tool in advancing precision medicine, offering the healthcare industry the ability to diagnose and treat complex conditions with greater confidence and efficiency.
In this article, we discuss the ins and outs of AI in this area of healthcare, including key applications, benefits, challenges (with potential solutions), and the role of cost-effective cloud infrastructure in making AI medical image analysis possible.
Uncover the latest trends in AI cloud computing and how to leverage the power of AI.Ebook: Navigating AI Cloud Computing Trends
Key Applications of AI-Based Image Analysis in Medicine
By leveraging advanced algorithms and deep learning technologies, AI in medical image analysis enhances the accuracy and efficiency of image analysis across various medical fields. From detecting early-stage diseases to aiding in personalized treatment plans, AI-based image analysis is shaping the future of diagnostics.
Below are the key applications of AI in medical image analysis and how they are revolutionizing patient care.
1. Radiology and Oncology
AI-driven image analysis is transforming radiology by enabling faster and more accurate detection of conditions such as tumors, fractures, and other abnormalities. Through the automated review of X-rays, MRIs, and CT scans, AI systems can identify even subtle signs of disease progression. This precision helps radiologists in diagnosing conditions earlier, supporting better patient outcomes and streamlining workflows in oncology and radiology departments.
2. Cardiology
In cardiology, AI-powered tools analyze heart scans like echocardiograms and cardiac MRIs to detect cardiovascular diseases. These medical image analysis AI tools can automatically identify signs of heart conditions, such as arrhythmias, valve defects, and signs of heart failure, by processing complex imaging data. This capability not only improves diagnostic accuracy but also aids cardiologists in monitoring treatment progress and making more informed decisions.
3. Ophthalmology
AI-based image analysis in ophthalmology is pivotal for diagnosing retinal diseases, such as diabetic retinopathy, macular degeneration, and glaucoma. By processing high-resolution images of the retina, AI systems can detect early signs of these conditions, often before symptoms manifest. This early detection supports timely intervention and effective management of retinal diseases, helping preserve patients’ vision.
4. Pathology
In pathology, image analysis AI enhances the analysis of biopsy samples and tissue imaging, speeding up diagnostic processes. AI models trained on vast data sets can identify patterns and markers indicative of cancer, infections, and other diseases in tissue samples. This capability not only accelerates diagnosis but also provides pathologists with detailed insights that contribute to more accurate, evidence-based diagnoses, significantly impacting patient care and treatment plans.
5. Dermatology
AI plays a crucial role in dermatology by leveraging advanced image analysis tools to enhance diagnostic accuracy and efficiency. Key benefits and applications include:
- Detection of Skin Conditions: AI tools analyze skin images to identify conditions like melanoma, psoriasis, and eczema with precision.
- Skin Cancer Screening: Advanced AI systems evaluate high-resolution images to detect signs of skin cancer, including subtle changes in moles or lesions that may go unnoticed by the human eye.
- Early Detection and Diagnosis: AI enables faster and more accurate diagnoses, supporting dermatologists in identifying conditions early and improving treatment outcomes.
- Standardized Evaluations: AI provides consistent assessments, reducing diagnostic variability and ensuring a standardized approach to monitoring skin health.
By integrating AI into dermatology, healthcare providers can enhance patient care, streamline workflows, and achieve better outcomes in skin health management.
6. Neurology
Image analysis AI is revolutionizing neurology by providing advanced tools to analyze brain imaging data, improving the diagnosis and management of neurological disorders. Key applications include:
- Detection of Neurological Disorders: AI assists in identifying conditions such as Alzheimer’s disease, Parkinson’s disease, epilepsy, and multiple sclerosis with precision.
- Advanced Scan Analysis: AI systems process complex imaging data, including MRIs and CT scans, to detect:
- Structural changes in the brain
- Tissue abnormalities
- Patterns linked to neurodegenerative conditions
- Early Detection and Intervention: By identifying abnormalities early, AI enables neurologists to intervene sooner, improving patient outcomes and supporting personalized treatment plans.
- Monitoring Disease Progression: AI tools track changes over time, helping neurologists assess disease progression and adjust treatment strategies accordingly.
- Evaluation of Therapeutic Effectiveness: AI aids in analyzing the impact of therapeutic interventions, ensuring treatments are optimized and effective.
Through these capabilities, AI enhances the accuracy, efficiency, and quality of neurological care.
Benefits of AI Image Analysis in Healthcare
As AI technology continues to advance, its role in healthcare will grow, providing support for increasingly complex diagnostic needs and driving new standards in patient care. Here are some important benefits of the technology:
1. Enhanced Accuracy
AI-based image analysis tools are designed to identify patterns and abnormalities with precision, supporting healthcare providers in catching even subtle early signs of disease that may be missed by human eyes. This accuracy is especially valuable in fields like radiology and oncology, where minor anomalies in imaging scans could indicate early-stage cancer, potentially improving patient outcomes through earlier interventions. Moreover, AI algorithms continuously learn and improve with increased data input, evolving their accuracy rates over time. By minimizing human error, AI helps reduce instances of misdiagnosis and ensures a more consistent standard in medical imaging interpretation.
2. Increased Efficiency
The diagnostic speed that AI brings to image analysis is transformative, particularly in time-sensitive medical cases such as trauma care, stroke, and cardiac emergencies. AI can rapidly analyze images and provide real-time or near-real-time insights to assist doctors in making critical decisions faster. This expedited process reduces the need for time-intensive, manual review, allowing radiologists and other medical professionals to handle more cases efficiently. For instance, automated analysis of chest X-rays for pneumonia or brain scans for stroke indicators can provide preliminary results much faster, enabling swift treatment decisions that could save lives.
3. Scalability in Screening
AI technology enables large-scale screening programs by automating the image analysis process, which is essential in areas with limited healthcare professionals or high patient volumes. For example, AI algorithms are used in tuberculosis and diabetic retinopathy screenings, allowing healthcare systems to conduct mass screenings efficiently. This scalability is particularly impactful in remote and underserved communities where access to specialized healthcare professionals, like radiologists, may be limited. In these areas, AI-based screening offers a practical, accessible solution, helping to detect conditions that would otherwise go unnoticed until they reach advanced stages.
4. Cost-Effectiveness
The integration of AI in medical imaging can help lower costs associated with the diagnostic process. Automated image analysis reduces the need for repeated imaging due to human oversight and supports more accurate initial diagnoses, potentially reducing follow-up consultations and additional testing costs. Moreover, AI can help manage the growing demand for medical imaging services without proportional increases in workforce and equipment investments. In the long run, AI-driven efficiencies in workflow and diagnosis reduce operational costs, making quality imaging more affordable and accessible within healthcare systems.
5. Augmentation of Clinician Capabilities
Rather than replacing human expertise, AI acts as an enhancement, providing healthcare professionals with critical insights and freeing up time for more complex tasks. This augmentation is particularly helpful in radiology, where AI can pre-screen images for certain conditions, flagging potential issues for further review by specialists. Clinicians can then focus on interpreting more complex cases and providing patient care. AI’s role as an assistive tool helps reduce clinician burnout by easing workloads, leading to improved morale and higher-quality care.
6. Standardized Diagnostic Protocols
AI can assist healthcare facilities in standardizing diagnostic protocols across various branches and locations, ensuring that all patients receive the same high level of care regardless of where they are. Through AI, smaller clinics and hospitals can adopt standardized imaging practices without the need for highly specialized staff, helping to bridge gaps between urban and rural healthcare services. This consistency leads to uniform quality of care, reducing variability in diagnostic outcomes, which is critical for chronic disease management and monitoring.
7. Enhanced Data Utilization and Research
AI systems used in image analysis gather extensive data over time, creating a valuable dataset for future research. These data can be anonymized and used for training algorithms, developing new diagnostic tools, and studying trends in disease progression or treatment outcomes. AI’s role in creating large datasets not only contributes to scientific understanding but also fuels innovations in medical technology, laying the groundwork for continuous advancements in healthcare.
How AI Algorithms Enhance Medical Image Analysis
AI algorithms are transforming the way medical images are analyzed, bringing unprecedented precision and efficiency to disease detection and diagnosis. Here’s a closer look at some of the core components that make AI-based medical image analysis so effective:
AI Algorithm Component | Description | Key Benefits |
Deep Learning Models | Uses convolutional neural networks (CNNs) to process complex image data, analyzing pixel-level details to identify structures and variations within medical images. | High accuracy in detecting complex patterns in X-rays, MRIs, CT scans, and other imaging modalities for early disease detection. |
Pattern Recognition | Recognizes and classifies specific patterns in medical images, which aids in identifying disease indicators such as tumors, blood vessel narrowing, or lung calcifications. | Enhances diagnostic accuracy, enabling early detection of abnormalities for proactive healthcare and timely intervention. |
Data Labeling and Training | Involves tagging specific features within images (e.g., tumors, fractures) to train AI models, improving their image interpretation and generalization across new cases. | Increased accuracy and reliability in diagnostics as AI models are trained on diverse, high-quality labeled datasets, refining AI’s disease recognition skills. |
Deep Learning Models
At the heart of AI-driven medical image analysis are deep learning models, particularly convolutional neural networks (CNNs), which excel at handling complex visual data. These neural networks process medical images by analyzing pixel-level details, identifying structures, and understanding variations that may be too subtle for human detection. CNNs are especially adept at recognizing intricate patterns within X-rays, MRIs, CT scans, and other imaging modalities. By segmenting and analyzing different regions within images, deep learning models can differentiate between healthy and abnormal tissues, making them invaluable for diagnosing conditions like tumors, fractures, or organ damage with high accuracy.
Pattern Recognition
AI’s ability to recognize and classify patterns in medical images is critical for early disease detection. By analyzing vast amounts of imaging data, AI algorithms learn to identify indicators associated with specific diseases—such as the irregular shape of a tumor, the narrowing of blood vessels, or calcifications in the lungs. This pattern recognition enables AI to detect abnormalities in their earliest stages, often before symptoms are visible or measurable. For example, in oncology, AI models trained to recognize the unique patterns of various cancers can highlight areas of concern in mammograms or lung scans, allowing for timely intervention. This capability enhances diagnostic accuracy and allows for more proactive, preventive healthcare.
Data Labeling and Training
For AI models to deliver accurate and reliable results, they require extensive labeled data to learn from. Data labeling involves tagging specific features within medical images (e.g., identifying tumor locations or types of fractures), which helps the AI recognize these features in future analyses. High-quality labeled data is crucial for training AI models, as it provides the foundation for learning and refining their image interpretation skills. The more accurately an AI model is trained with diverse, labeled datasets, the more proficient it becomes at generalizing across new cases, leading to more reliable diagnostic outcomes. Medical institutions and AI companies are increasingly collaborating on data labeling initiatives to improve the accuracy and relevance of AI algorithms in image analysis.
Challenges in AI Medical Image Analysis
While AI has shown tremendous promise in medical image analysis, several challenges remain to ensure its successful and ethical integration into healthcare.
Challenge | Why it’s a Challenge | Solution |
Data Privacy and Security | Ensuring patient data remains secure and complies with regulations like HIPAA and GDPR. | Implement strong encryption, anonymization, and access control measures. |
Bias in AI Models | AI models may produce biased results if trained on non-representative datasets. | Use diverse datasets to train AI models, ensuring inclusivity across demographics. |
Interpretability and Validation | AI systems often lack transparency, making it difficult for physicians and regulators to trust outputs. | Develop interpretable models and establish clear validation processes for regulatory approval and clinical trust. |
Integration with Existing Systems | Integrating AI into legacy healthcare infrastructures can disrupt workflows. | Ensure compatibility with existing systems like EHRs and PACS, and streamline adoption with user-friendly tools. |
Regulatory and Ethical Considerations | Lack of established regulatory frameworks for AI creates hurdles in validation and accountability. | Establish clear regulatory standards and address ethical concerns about responsibility for AI-driven decisions. |
Cost and Resource Barriers | Significant investment and resources are needed for AI development and deployment. | Develop scalable, affordable AI solutions to enable broader adoption, especially in resource-limited settings. |
1. Data Privacy and Security
AI relies on large volumes of patient data, which raises concerns about privacy and security. Ensuring that patient data remains confidential and is securely processed by AI algorithms is crucial for compliance with regulations like HIPAA and GDPR. Establishing strong encryption, anonymization, and access control methods is essential to maintaining trust in AI systems used for sensitive health information.
2. Bias in AI Models
Bias in AI models is a major issue that can lead to inaccuracies in diagnoses, especially if the AI is trained on data that lacks diversity. A dataset that isn’t representative of various patient demographics, including age, race, and sex, can result in biased AI outputs that impact healthcare equity. To improve accuracy and inclusivity, it’s important to develop AI models trained on diverse datasets, which can better generalize to different patient populations.
3. Interpretability and Validation
For AI systems to gain the trust of physicians and regulators, their results need to be interpretable and thoroughly validated. Medical professionals require transparency in AI decision-making processes to understand and verify findings, especially for regulatory approval. Developing models that not only achieve high accuracy but also provide explanations for their decisions is crucial for building trust and securing approval for clinical use.
4. Integration with Existing Systems
Integrating AI software into existing healthcare systems and workflows can be complex. Healthcare infrastructures often rely on legacy systems that aren’t easily compatible with modern AI applications. Ensuring that AI solutions can seamlessly integrate with existing technologies, such as electronic health records (EHRs) and PACS systems, is essential for smooth adoption, minimizing disruption, and optimizing the benefits of AI in medical settings.
5. Regulatory and Ethical Considerations
The rapid advancement of AI in healthcare has outpaced regulatory frameworks, leaving gaps in standards for AI implementation. Navigating the regulatory approval process for AI-driven medical imaging tools is challenging due to a lack of universally accepted benchmarks for validation and safety. Additionally, ethical concerns surrounding the responsibility for AI-driven decisions must be addressed to ensure accountability in clinical outcomes.
6. Cost and Resource Barriers
Developing and deploying AI-based medical imaging solutions requires significant financial investment, technical expertise, and computational resources. Many healthcare facilities, especially in resource-limited settings, face challenges in acquiring the infrastructure necessary to implement these technologies. Addressing cost barriers through scalable, affordable AI solutions will be critical for widespread adoption and equitable access.
NZO Cloud’s Role in Providing the Foundation for AI Medical Image Analysis
When it comes to AI medical image analysis, the first thing that a healthcare organization needs is a robust cloud environment with solid infrastructure. On-premises solutions were once a fair possibility, as the amount of data, while significant, could still be processed with relatively affordable hardware and software. However, the landscape when it comes to data and, subsequently, AI (which obviously relies on data to be effective) has changed dramatically. The rise of big data, and the need for management of increasingly large data sets requires a significant investment in infrastructure that makes it unrealistic to rely on on-premises hardware or rented space in data centers. Instead, cloud-based infrastructure is essential, and big players like Amazon, Google, and Microsoft have more than enough of it to provide organizations with the computational power they need.
However, the costs typically associated with high-performance cloud computing can quickly spiral out of control, as there are so many factors to consider, including regional data transfer, bandwidth, and processing requirements. This means that significant time needs to be spent calculating and estimating costs using the cost calculator tools these services provide. But even with that time investment, varying workloads can easily tip the scales and cause healthcare organizations to go over budget.
With NZO, however, healthcare organizations get full control over their cloud performance with the peace of mind that they won’t exceed their budget. You get to build your customized cloud infrastructure, complete with the security and design that you need to manage your data effectively. In turn, this allows more focus to be put on AI medical analysis and less on the backend.
- Fixed subscription pricing gives healthcare organizations full control over their budget while ensuring they still have the cloud performance they need
- NZO provides full onboarding to ensure that users are ready to use the system to its fullest capabilities
- Security engineering teams are available to help you ensure you have the right data privacy and other security measures in place in your cloud infrastructure
Conclusion
AI-driven medical image analysis is revolutionizing healthcare by enhancing diagnostic accuracy, streamlining workflows, and expanding access to life-saving technologies. From early disease detection to personalized treatment plans, AI has demonstrated its potential to significantly improve patient outcomes while reducing costs and increasing efficiency. Despite challenges like data privacy, model bias, and integration hurdles, the advancements in AI and cloud-based solutions signal a promising future for this field.
As healthcare organizations embrace AI, the role of robust cloud infrastructure, such as NZO’s secure and cost-effective platform, becomes critical. By providing scalable, customizable, and budget-friendly solutions, platforms like NZO ensure that medical professionals can focus on leveraging AI for better diagnostics without worrying about backend complexities.
Gain control over your cloud environment with NZO. Reach out to us today for a free trial.