Table of Contents
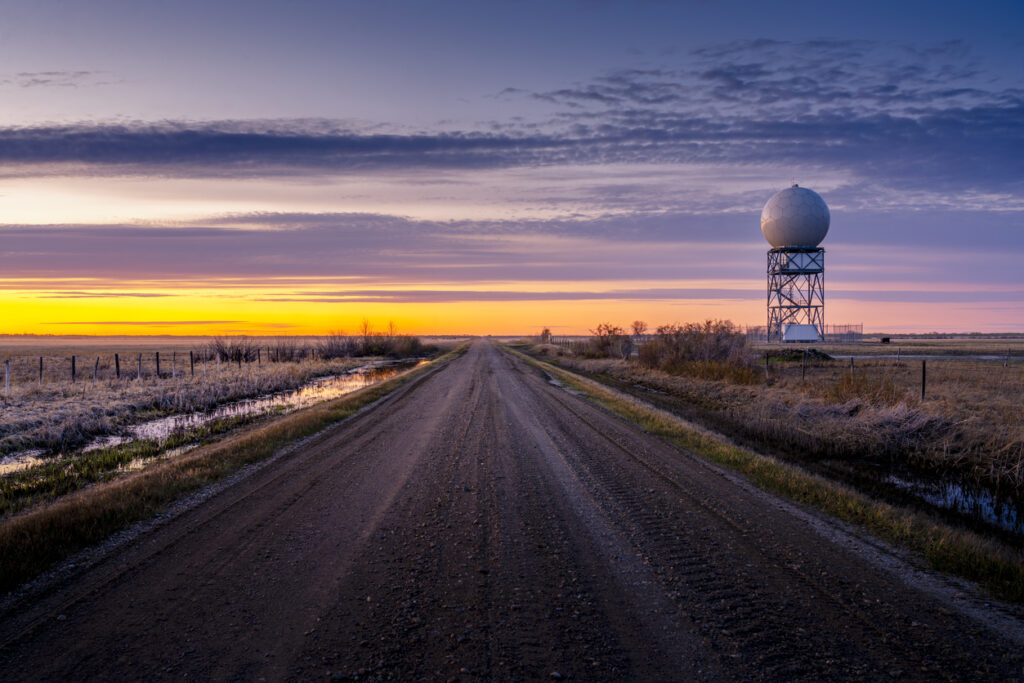
Artificial intelligence (AI), powered by high-performance computing, has been proven to be a game-changer in weather prediction, revolutionizing how industries, governments, and communities prepare for and respond to atmospheric changes. By harnessing advanced algorithms, machine learning, and vast datasets, AI-driven weather forecasting offers unprecedented accuracy, efficiency, and adaptability.
From disaster preparedness and agriculture to aviation, urban planning, and beyond, AI transforms traditional approaches to weather prediction, enabling data-driven decisions that save lives, protect property, and optimize operations. However, as AI’s influence grows, so do the challenges and ethical considerations tied to its application.
This article delves into the applications, benefits, risks, and future trends of AI in weather prediction, offering a comprehensive look at its potential and limitations.
The importance of HPC in weather modeling, emerging trends, and the potential for AI.Ebook: Cloud Computing for Weather Modeling
Applications and Benefits of AI Weather Prediction
AI weather prediction is transforming how industries and governments prepare for and respond to weather events. By leveraging advanced algorithms and massive datasets, AI offers precision and efficiency in weather forecasting that traditional methods cannot match. Here’s how AI weather prediction is making an impact across various sectors:
1. Disaster Preparedness
AI for weather prediction plays a crucial role in mitigating the impacts of extreme weather events such as hurricanes, tornadoes, floods, and wildfires.
- Real-Time Forecasting: AI models analyze massive datasets from the cloud, including satellite imagery and historical weather patterns, to deliver highly accurate forecasts in real-time. These forecasts enable agencies to predict the path, intensity, and timeline of severe weather events.
- Resource Allocation: Emergency response teams can strategically position supplies, personnel, and evacuation centers based on AI forecasts. For instance, predicting the trajectory of a hurricane days in advance allows governments to minimize casualties and infrastructure damage.
- Flood Risk Mapping: AI combines rainfall data with topographical information to predict which areas are at high risk of flooding, enabling proactive flood defenses.
2. Agriculture and Farming
The agricultural sector relies heavily on weather conditions, and AI weather prediction helps farmers optimize their operations and reduce losses.
- Precision Agriculture: AI tools provide hyper-local forecasts, guiding farmers on the best times to plant, water, and harvest crops. Predicting an early frost can prevent significant crop losses.
- Pest and Disease Management: AI predicts weather conditions that favor the spread of pests or crop diseases, allowing farmers to take preventive measures.
- Water Resource Optimization: By forecasting rainfall and evaporation rates, AI helps farmers manage irrigation systems more efficiently, conserving water while maintaining crop health.
- Climate Adaptation: Farmers can use AI insights to select crop varieties and planting schedules better suited to changing weather patterns, ensuring long-term sustainability.
3. Aviation and Shipping
Safety and efficiency are paramount in the aviation and maritime industries, and weather prediction using AI significantly enhances both.
- Safer Routes: AI provides real-time updates on weather conditions, helping airlines and shipping companies avoid severe storms, turbulence, and high seas. This reduces the risk of accidents and improves passenger and cargo safety.
- Fuel Efficiency: By identifying optimal routes with favorable wind and ocean current conditions, AI helps reduce fuel consumption and operational costs. For example, predicting tailwinds allows airlines to adjust flight paths for faster travel.
- Delay Reduction: AI can forecast adverse weather conditions that might disrupt schedules, enabling airlines and shipping companies to make preemptive changes to minimize delays and logistical challenges.
4. Public Safety and Urban Planning
AI in weather prediction provides municipalities and urban planners with actionable insights to design safer, more resilient cities.
- Infrastructure Resilience: AI predicts weather-related risks such as floods, heatwaves, or heavy snowfall, helping cities design infrastructure to withstand these challenges. For instance, AI can recommend locations for stormwater management systems or heat-resistant road materials.
- Heatwave Preparedness: Cities can use AI forecasts to plan for heatwaves by opening cooling centers and issuing public health advisories.
- Emergency Services: AI-driven weather models inform local authorities of potential hazards, allowing them to deploy police, fire, and ambulance services strategically.
- Pollution Management: AI can integrate weather data with pollution forecasts, helping cities issue air quality warnings and implement measures to reduce emissions during adverse conditions.
5. Renewable Energy Management
The growing renewable energy sector benefits immensely from AI-driven weather forecasting to optimize energy production and distribution.
- Wind Energy: AI predicts wind speeds and directions to optimize the operation of wind turbines, maximizing energy generation and minimizing wear and tear on equipment.
- Solar Power: By forecasting cloud cover and sunlight hours, AI helps solar farms plan energy storage and manage supply to the grid effectively.
- Energy Grid Stability: AI forecasts assist energy companies in balancing supply and demand by predicting weather-driven fluctuations, ensuring uninterrupted power distribution.
6. Insurance and Risk Assessment
AI weather prediction helps the insurance industry mitigate risks and plan for extreme weather events.
- Risk Modeling: Insurers can use AI to assess the probability of weather-related claims, such as those stemming from hurricanes or floods, enabling better risk management.
- Customized Policies: AI-driven forecasts allow insurers to tailor policies based on regional weather risks, offering more accurate premiums and coverage options.
- Claims Processing: In the aftermath of weather-related disasters, AI can assist in processing claims quickly by cross-referencing real-time weather data with policyholder locations.
7. Tourism and Event Planning
AI weather prediction enables businesses in the tourism and event planning sectors to make data-driven decisions that enhance customer experience and minimize disruptions.
- Seasonal Planning: Resorts and outdoor event organizers can adjust their schedules and services based on accurate long-term weather forecasts, ensuring peak customer satisfaction.
- Real-Time Adjustments: AI predictions allow event managers to adapt to sudden weather changes, such as shifting outdoor activities indoors or rescheduling based on rainfall or extreme heat.
By leveraging AI-driven weather insights, industries and governments can anticipate challenges, optimize operations, and safeguard communities, creating a more resilient and sustainable future. Weather prediction using AI is not just for understanding the environment; it is a strategic advantage for thriving within it.
Potential Dangers of Leaving Weather Prediction to AI
While AI has revolutionized weather prediction with its accuracy and speed, relying exclusively on AI models without human oversight introduces significant risks. Understanding these potential dangers is essential to ensure AI’s role in weather forecasting remains balanced and effective.
1. Over-Reliance on AI Models
AI systems are powerful tools, but fully depending on their predictions without human oversight can lead to critical mistakes.
- Human Context: Weather forecasting often requires interpreting subtle environmental cues and anomalies that AI may not recognize. Without human meteorologists to validate results, AI-generated forecasts could overlook critical details.
- Decision-Making Risks: Sole reliance on AI predictions could result in automated decisions that fail to account for nuances, such as local terrain effects or community-specific vulnerabilities.
2. Model Limitations and Error Margins
AI weather prediction models are only as good as the data and algorithms they rely on, which means inaccuracies in inputs can lead to flawed predictions.
- Incomplete Data: AI predictions often depend on real-time data from satellites, sensors, and historical records. Missing or outdated data can skew results, particularly in regions with sparse monitoring networks.
- Error Margins: Even advanced AI models have error margins, and small inaccuracies in predicting severe weather events like hurricanes or tornadoes could have devastating consequences for communities.
- Edge Cases: AI may struggle with rare or unprecedented weather patterns that fall outside its training data, leading to potentially incorrect forecasts.
3. Algorithmic Bias
AI models are prone to bias if trained on incomplete or unbalanced datasets.
- Data Imbalance: Training AI models primarily on data from well-monitored regions could lead to reduced accuracy for predictions in underserved areas, such as remote or developing regions.
- Misinterpretation of Outliers: AI may misclassify unusual weather patterns as errors or fail to recognize them as indicators of rare events, resulting in missed warnings or false alarms.
4. Lack of Interpretability
Many AI systems function as “black boxes,” making it challenging to understand how predictions are generated.
- Trust Issues: Without clear explanations of how AI models arrive at conclusions, meteorologists and decision-makers may struggle to trust or validate predictions.
- Challenges in Public Communication: Explaining complex AI-driven forecasts to the public can be difficult, potentially undermining trust in weather warnings or advisories.
5. Vulnerability to Cyber Threats
AI systems rely on vast networks of data, making them susceptible to cyberattacks.
- Data Tampering: Hackers could manipulate real-time data inputs to disrupt predictions, causing false alarms or delays in critical warnings.
- Infrastructure Risks: Attacks on cloud-based AI systems could compromise entire forecasting networks, leaving regions without reliable predictions during emergencies.
6. Ethical and Accountability Concerns
Fully automating weather prediction raises ethical questions about responsibility.
- Who is Accountable?: If an AI system makes an incorrect prediction leading to loss of life or property, it’s unclear whether responsibility lies with the technology provider, developers, or organizations using the system.
- Automation Fatigue: Over-reliance on AI could lead to reduced investment in human meteorologists and expertise, making it harder to maintain balanced systems in the long run.
Ethical and Responsibility Concerns in AI Weather Prediction
As AI becomes more central to weather forecasting, significant ethical and responsibility concerns arise. These challenges focus on accountability, transparency, and the interplay between human expertise and AI-driven automation. Balancing these elements is crucial to ensure accurate forecasts, public safety, and equitable disaster preparedness.
Concern | Why It’s a Concern | Key Issues |
Accountability | Responsibility for AI-driven forecasting failures is unclear. | Challenges include identifying whether developers, deploying organizations, or interpreters are liable. |
Transparency | Stakeholders must understand how AI generates predictions. | Essential for informed and verifiable decision-making. |
AI vs. Human Expertise | AI excels in processing data, but humans provide critical interpretation. | Over-reliance on AI risks eroding human meteorological expertise. |
Bias in Models | AI models may perpetuate bias from incomplete or unrepresentative historical data. | Can lead to disparities in forecasting accuracy and disaster preparedness. |
Data Limitations | AI performance depends on the availability and quality of input data. | Limited or poor data from remote or underserved areas may skew predictions. |
Public Trust | Errors in predictions can erode confidence in AI-driven systems. | Open communication is needed to maintain trust and clarify AI’s limitations and strengths. |
Communication of Uncertainty | AI lacks the nuanced communication that human meteorologists provide. | Miscommunication may lead to undue panic or complacency. |
1. Accountability in AI Predictions
Determining accountability in AI-driven forecasts can be complex, particularly when predictions fail or lead to inadequate disaster preparedness. Questions arise about whether responsibility lies with the developers of the AI models, the organizations deploying them, or the individuals interpreting the results. This lack of clarity can complicate responses to errors and undermine trust in AI systems. Transparency in AI models is equally critical; stakeholders must understand how predictions are generated to ensure decisions are informed and verifiable.
2. The Balance Between AI and Human Expertise
AI offers unmatched efficiency in processing large datasets, but human meteorologists bring invaluable contextual understanding to forecasts. While AI may detect intricate patterns, humans interpret these findings within broader environmental and social contexts, ensuring actionable and meaningful predictions. However, over-reliance on AI could diminish the role of meteorologists, leading to a gradual erosion of human expertise. Maintaining a balance between AI’s computational power and human interpretative skills is vital for accurate and reliable forecasting.
3. Bias and Data Limitations
AI models often rely on historical data, which can introduce bias if the data is incomplete or unrepresentative. Such biases may lead to inaccurate predictions, especially for regions or populations historically underrepresented in weather datasets. Additionally, AI’s performance hinges on the quality and availability of data. Inadequate or low-quality data, particularly in remote or underserved areas, can result in skewed forecasts, leaving vulnerable populations insufficiently prepared.
4. Public Trust and Communication Challenges
AI predictions often lack the nuanced communication of uncertainty that human meteorologists provide. This absence can lead to misunderstandings about the reliability of forecasts, causing either unnecessary panic or unwarranted complacency. Furthermore, errors in AI predictions may erode public trust in weather forecasting systems. To maintain confidence, it is essential to communicate openly about the limitations and strengths of AI-driven forecasts while emphasizing their role in improving public safety.
Future Trends in AI and Weather Prediction
The evolution of AI in weather forecasting is set to revolutionize how we understand and predict atmospheric changes. By integrating cutting-edge technologies and methodologies, the future of weather prediction looks more precise, adaptive, and impactful than ever before. Here are key trends shaping the future of AI in weather forecasting:
Trend | Description |
Hybrid Forecasting Models | Combines traditional methods with AI for balanced, reliable weather predictions, leveraging AI for speed and traditional models for validation. |
Advanced Sensors and IoT | IoT devices and advanced sensors collect hyperlocal data, enabling AI to improve forecasting accuracy and cover remote or inaccessible regions. |
Long-Term Climate Predictions | AI analyzes historical data to model climate trends and assess impacts of policy decisions, aiding in sustainable development and climate change mitigation. |
Real-Time Forecast Adjustments | AI dynamically updates forecasts for rapidly changing conditions, providing real-time alerts for emergencies like hurricanes or heatwaves. |
Global Collaboration | AI platforms facilitate global data sharing, enhancing forecasting accuracy and extending capabilities to underrepresented and remote regions. |
Hybrid Forecasting Models
Hybrid weather forecasting models, which combine traditional meteorological methods with AI-driven predictions, are emerging as a cornerstone of advanced weather forecasting. Traditional models excel at capturing physical processes and understanding the nuances of regional climates, while AI provides unparalleled speed and computational power to analyze vast datasets. By blending these approaches, hybrid models offer a more balanced and reliable forecasting solution. For example, while AI might quickly identify a trend or anomaly, traditional physics-based models can validate these findings to ensure they align with known atmospheric behaviors. This fusion minimizes the risk of over-reliance on AI, offering decision-makers more accurate and actionable insights.
Advanced Sensors and IoT Integration
The integration of Internet of Things (IoT) devices and advanced sensors is revolutionizing how weather data is collected and utilized. Modern IoT devices such as drones, satellite systems, and ground-based weather stations are capable of providing real-time, hyperlocal data on critical factors like temperature, wind speed, humidity, and air pressure. AI processes this influx of data to generate highly detailed and localized forecasts. For instance, in smart cities, IoT-based systems can deliver tailored predictions for specific urban districts, aiding traffic management, energy grid optimization, and emergency response planning.
In addition, new technologies such as LiDAR (Light Detection and Ranging) and radar-equipped drones are opening up data collection possibilities in previously inaccessible regions, such as mountainous terrains or over oceans. This increased breadth of data enables AI models to improve forecasting accuracy, particularly in regions prone to extreme or sudden weather changes.
Long-Term Climate Predictions
AI’s capabilities are proving invaluable in modeling and predicting long-term climate trends. By analyzing decades of historical data, satellite imagery, and current environmental patterns, AI-driven systems can provide insights into the impacts of climate change. These predictions are crucial for understanding trends like rising global temperatures, melting polar ice caps, and the increased frequency of extreme weather events such as hurricanes and droughts.
AI also enables climate models to simulate future scenarios based on various policy interventions, such as emission reductions or renewable energy adoption.
Policymakers can use these simulations to evaluate the potential outcomes of different strategies and design actionable plans to combat climate change. For instance, AI-based models might project the benefits of reforestation in mitigating carbon emissions, offering data-driven support for sustainable development initiatives.
Real-Time Forecast Adjustments
AI systems are increasingly capable of making real-time adjustments to weather forecasts as new data becomes available. This dynamic approach ensures that predictions remain up-to-date, even for rapidly changing weather conditions like hurricanes or flash floods. Real-time forecasting is particularly valuable for critical situations, enabling governments and emergency response teams to react swiftly and effectively.
In addition to improved predictions, AI-driven systems can also deliver personalized weather alerts to individuals through mobile apps and public warning systems. For example, an AI-powered weather service might analyze a user’s location and provide tailored notifications about incoming storms, heatwaves, or other hazards, allowing people to prepare accordingly.
Global Collaboration Through AI Platforms
AI-powered cloud platforms are fostering greater global collaboration among meteorological agencies and researchers. By enabling the sharing of weather data, models, and insights across borders, these platforms enhance forecasting accuracy on a worldwide scale. For instance, a cloud-based system might pool data from satellites operated by different nations, creating a unified database for more comprehensive analysis.
This global collaboration is particularly important for addressing challenges like monitoring polar weather conditions, which are difficult to study due to their remoteness, and improving forecasting capabilities in underrepresented regions like parts of Africa or Southeast Asia. By creating a shared data ecosystem, AI platforms help ensure that no region is left behind in the quest for accurate and actionable weather predictions.
Uncover the latest trends in AI cloud computing and how to leverage the power of AI.Ebook: Navigating AI Cloud Computing Trends
Conclusion
AI-driven weather prediction represents a significant leap forward in forecasting accuracy, efficiency, and responsiveness. Its applications across disaster management, agriculture, aviation, and urban planning demonstrate its ability to enhance decision-making and improve preparedness for both everyday weather and extreme events. While the technology offers immense promise, it also presents risks such as over-reliance on AI models, algorithmic bias, and data limitations, underscoring the need for a balanced integration of AI and human expertise.
Looking ahead, the future of AI in weather prediction is bright, marked by innovations like hybrid forecasting models, IoT integration, real-time adjustments, and global collaboration through shared platforms made possible by the cloud. These advancements not only promise greater precision but also pave the way for long-term climate solutions, addressing critical global challenges. As AI continues to evolve, its success will hinge on ethical responsibility, robust data quality, and fostering trust among stakeholders to ensure that technology serves as a tool for resilience and progress in an ever-changing climate landscape.
Take control of your cloud environment with NZO. Reach out to us today for a free trial.